Invasive Species
This use case (UC) covers Invasive Alien Species. Together with the Endangered Species UC, it will populate and demonstrate the 'Dynamics and Threats from and for Species of Policy Concern' part of the Biodiversity Digital Twin. At present, the Endangered Species UC has not yet been developed.
This UC aims at quantifying the levels of invasion (i.e., the number of naturalized alien species) in different terrestrial habitat types across Europe under baseline conditions and various future climate and land-use change scenarios. The primary outcome of the use case will be spatially explicit and habitat-explicit projections of current and future invasion levels that can be updated as new current species distributions and/or scenario projections of environmental data become available. The UC will first explore naturalized alien plant species because of abundant vegetation and floristic data, which allow mapping species to habitat types. This will allow producing more refined estimates of invasion levels that are more informative for management and policy-making.
At the core of the Invasive Alien Species Digital Twin (IAS-DT) is joint species distribution modeling (JSDM). In the JSDM framework, the invasion level is calculated by modeling the presence of multiple co-occurring alien species and then summing species occurrences at a chosen spatial resolution. Compared to single-species or stacked species distribution models, JSDMs can better estimate the effects of environmental factors on species distributions because the information across many species is pooled together. Additionally, by leveraging the residual information, JSDMs can better predict species co-occurrence patterns and the overall assemblage composition. Such an approach is, therefore, advantageous to the previously employed approach to modeling the levels of plant invasion in Europe that involved first aggregating species occurrence data in calculating the level of invasion and then modeling the latter as a function of environmental predictors.
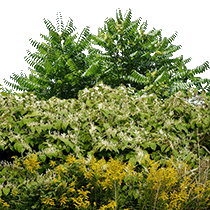
Invasive Species and Digital Twin Models
Data streams for the IAS-DT input data will be prepared per individual data source (i.e., per Research Infrastructure) and per data type (i.e., species occurrences, habitat, environment, etc.). A schema of the data streams can be seen in Figure 1 below.
Dynamic Data Driven Application Systems (DDDAS) is a conceptual framework that synergistically combines models and data in order to facilitate the analysis and prediction of physical phenomena. It is a two-layer system design where data assimilation and feedback loops create a bi-directional (data ßà model) information flow. These workflows will prepare data from the data sources in processing steps and will output the data into HDF5/netCDF4 files. Subsequent changes in data sources will be detected through feedback loops, and new data will be assimilated into the output files.
The raw output from JSDMs will be matrices of predicted species occurrence probability, which will then be post-processed into binary presence-absence matrices and the estimates of invasion levels (i.e., sums of individual species presences per grid cell per habitat). Once the models are parameterized on the baseline data, we will use them to produce projections under chosen climate and land-use (or land management) scenarios and visualize the levels of invasion (i.e., sums of species presences per grid cell) as maps.